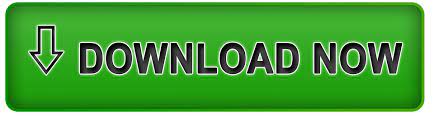
Nick Bostrom's 2014 book Superintelligence: Paths, Dangers, Strategies sketches out Good's argument in detail, while citing writing by Yudkowsky on the risk that anthropomorphizing advanced AI systems will cause people to misunderstand the nature of an intelligence explosion. Good, recursively self-improving AI systems quickly transition from subhuman general intelligence to superintelligent. In the intelligence explosion scenario hypothesized by I. In response to the instrumental convergence concern, where autonomous decision-making systems with poorly designed goals would have default incentives to mistreat humans, Yudkowsky and other MIRI researchers have recommended that work be done to specify software agents that converge on safe default behaviors even when their goals are misspecified.

Thus the challenge is one of mechanism design-to design a mechanism for evolving AI under a system of checks and balances, and to give the systems utility functions that will remain friendly in the face of such changes.
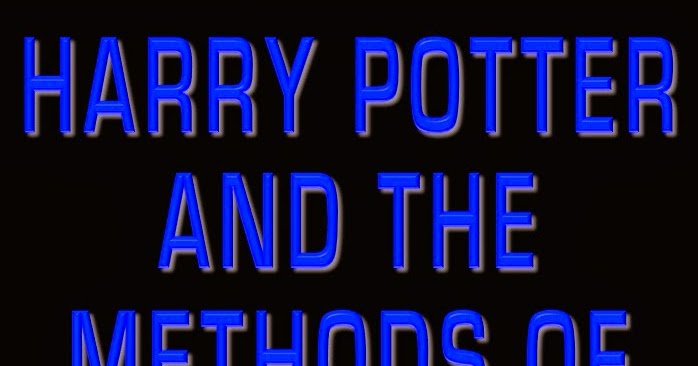
He asserts that friendliness (a desire not to harm humans) should be designed in from the start, but that the designers should recognize both that their own designs may be flawed, and that the robot will learn and evolve over time. Yudkowsky (2008) goes into more detail about how to design a Friendly AI.
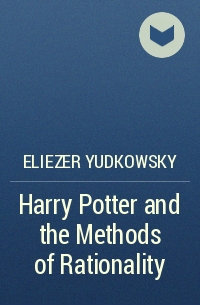
Noting the difficulty of formally specifying general-purpose goals by hand, Russell and Norvig cite Yudkowsky's proposal that autonomous and adaptive systems be designed to learn correct behavior over time: Yudkowsky's views on the safety challenges posed by future generations of AI systems are discussed in the undergraduate textbook in AI, Stuart Russell and Peter Norvig's Artificial Intelligence: A Modern Approach.
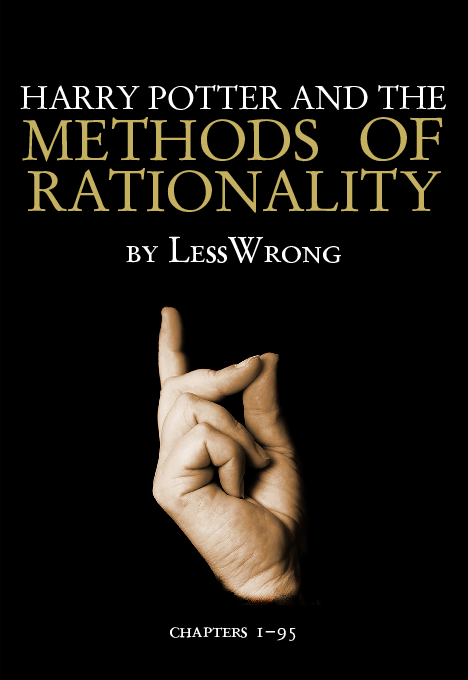
See also: Machine Intelligence Research Institute Goal learning and incentives in software systems
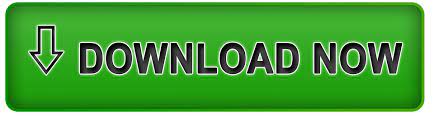